In today's digital era, big data has become a key resource for enterprises and organizations in decision-making, business optimization, market forecasting, etc. The basic process of big data processing involves multiple key steps, from data collection to final analysis and application. This article will delve into the basic processes of big data processing to help readers better understand how to effectively utilize this precious resource.
In order to understand the basic processes of big data, it is first necessary to understand its five defining characteristics:
1. Capacity (Volume):
Definition: The "capacity" characteristic of big data refers to the large scale of the data, which far exceeds the range that traditional data processing systems can handle.
Significance: Big data usually exists in massive amounts of data, which may involve tens to hundreds of terabytes or even more. Processing such large-scale data requires the use of distributed computing and storage systems such as Hadoop.
2. Variety:
Definition: "Diversity" means that big data can contain a variety of different types of data, including structured data, semi-structured data, and unstructured data.
Significance: Traditional relational databases mainly deal with structured data, while big data often involves various data types from different sources, such as text, images, audio, video, etc. This makes processing and analyzing the data more complex.
3. Velocity:
Definition: The "speed" feature emphasizes the speed of data generation, flow and processing, that is, the real-time requirement of data.
Significance: In some scenarios, data needs to be processed in real-time or near real-time, such as financial transactions, social media updates, etc. Big data systems need to have the ability to process and analyze data at high speed to cope with this rapidly changing data flow.
4. Veracity:
Definition: "Authenticity" means concern for the accuracy and trustworthiness of data, especially with regard to data quality.
Significance: Big data often contains data from different sources, which may be inconsistent, inaccurate or incomplete. Therefore, in the big data processing process, it is crucial to ensure the authenticity and quality of data to avoid analysis and decision-making on erroneous or unreliable data.
5. Value:
Definition: The "value" feature emphasizes the ability to extract useful information from big data, that is, the ultimate goal of data analysis is to create value.
Significance: The ultimate goal of big data processing is to gain meaningful insights into the business through in-depth analysis and mining. This helps businesses make smarter decisions, optimize operational processes, and discover new business opportunities.
The big data processing process includes four core stages:
1. Data Collection:
This stage involves collecting large amounts of primary data from multiple sources. Data can come from multiple sources such as sensors, logs, social media, business applications, and more. The type of data may be structured, semi-structured or unstructured.
2. Data Storage:
The collected data needs to be stored in a suitable storage system. Big data storage systems are usually distributed and capable of processing large-scale data. Common storage systems include distributed file systems (such as Hadoop's HDFS), NoSQL databases (such as MongoDB, Cassandra) and relational databases (such as MySQL, PostgreSQL).
3. Data Processing:
Data processing is the core of the big data processing process. At this stage, operations such as cleaning, transformation, and aggregation of stored data are performed to prepare for subsequent analysis. Big data processing frameworks such as Apache Spark and Apache Flink are widely used to efficiently process large-scale data.
4. Data Analysis and Application:
After data processing is completed, data analysis is performed to extract useful information. This may include statistical analysis, machine learning, data mining and other techniques. The results of the analysis can be used to formulate business strategies, predict trends, optimize business processes, etc. Ultimately, the results of analysis can be applied to business decisions, product improvements, etc.
The role of IP proxy in big data
IP proxies act as a middleman between the client and the target server, hiding the client's IP address and allowing anonymous access to data sources, which can be used in big data projects. By using IP proxies, web crawlers can bypass IP blocking, CAPTCHA and rate limiting, ensuring seamless data collection.
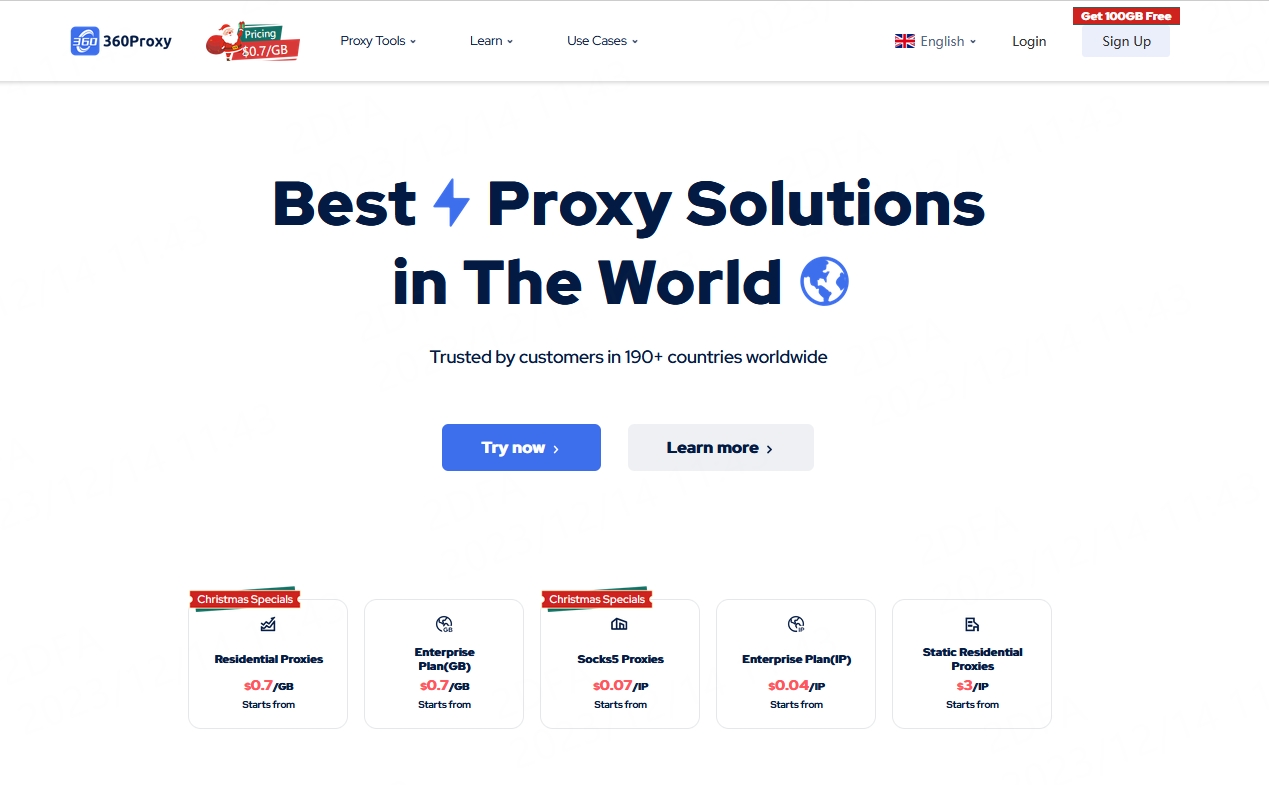
360Proxy is a professional proxy service provider. They offer a variety of residential and data center proxy solutions to meet the needs of businesses of all sizes, and their proxy services feature:
- High-performance proxy with low latency
- 99.9% uptime guarantee
- Easy integration with common web scraping tools
- Competitive price
In short, the basic process of big data revolves around the systematic collection, storage, processing and analysis of large amounts of information. IP proxies play a key role in achieving efficient data collection, and 360Proxy is a reliable proxy service provider worth recommending. By understanding these fundamental aspects, businesses can leverage the power of big data to drive innovation and gain a competitive advantage.